Guest Interview with Katie Fernandez
Katie is a data analyst with an educational background in math and in this episode she shares her tips on how to confidently communicate your data work. We also have a segment where she advises on how to present certain data terms.
You can also listen on Apple Podcasts, Google Podcasts, and Spotify.
What You’ll Learn in this episode
- Katie’s experience with communicating data as a data analyst
- Advice she has for new data professionals struggling
- How to “translate” and present certain data terms in your communications with a non-data audience
About Katie
I currently work in the healthcare industry and previously worked in the insurance industry. I found the data field through my love of math and after my first SQL course I was hooked! In my free time I love to spend time with my son- affectionately called “The Intern”- and play video games.
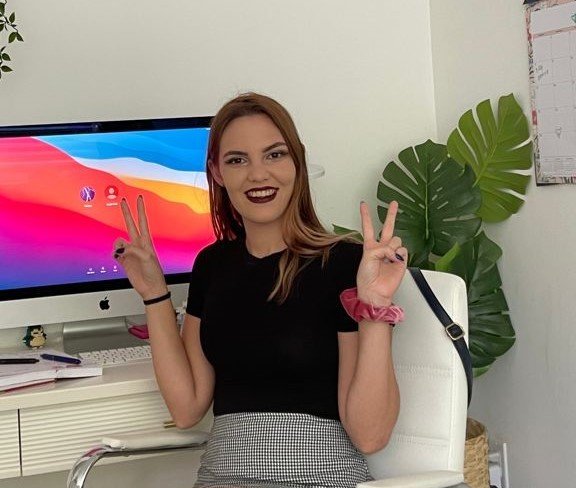
How to Connect with Katie
Instagram: KT.dbs
Tiktok: KT.dbs
Twitch: KTDBS
Check out her new website: https://uhhdata.com/
Get in Touch with Hana
Let me know what you think of the episode, you can message at hana@trending-analytics.com or on Instagram @hanalytx.
If you are looking for podcast updates and want additional tips on how to visualize and present data sent straight to your inbox, then make sure to subscribe to my weekly data letters here.
When you hit that subscribe button, I’ll be sliding into your inbox every Wednesday with an email.
Love the show? Why not leave a review?
If you loved this episode of the Art of Communicating Data Podcast, why not leave a review on Apple Podcasts and Spotify?
It only takes 2 minutes and provides me with invaluable insight as to what the listeners think.
If you enjoyed this episode, check out this episode where I share tips on ways you can improve your data visualizations.
Episode Transcript
So today we're joined by Katie Fernandez. She is a data analyst who currently works in the healthcare industry. She also has a son that she affectionately calls the intern. And if you follow her on Instagram, you would know that several of us have actually advocated for the intern to get a promotion a while back. Did he get a promotion? Um, I mean, he's going to school now. I guess that's kind of a promotion. Okay. Yeah. Fair enough. It counts. Yeah, that counts. What I find really interesting about your account is your love for math. And I really appreciate how you try to educate the rest of us who are not so good in math. You know, we're working in data, but we may not always be good at math. And so I really like how your videos, especially on Tik, talking on Instagram, you're trying to teach us. So could you tell us a little bit more about like how math brought you to the world of data. Absolutely. So I actually discovered that I was good at math in my sophomore year of high school, which was kind of late. And then when I graduated high school, I said, I want to do more of this. Like I just want to do calculus for the rest of my life. Um, and so I became a math major and, when you're a math major, it's not like one of those degree titles that says what your job is going to be. There is no person who does calculus all day, like they have a different job title. So it was looking into how to use my degree. And one of the things that popped up was data scientist, and that was very appealing. So I looked into it, I read more about it and I eventually decided that this was what I wanted to pursue. And I switched my degree out of math, into data analytics, and that was easily one of the best decisions I've made to date. But I still kept practicing math and algebra and all that through tutoring students between the ages of as young, as kindergarten to college level math. And that kept me sharpened that kept me focused on the math and it helped. That's really great to hear. And you are an official math tutor for all of us in the data community now. I'm so happy. I love that, how it brought you to the world of data, specifically data analytics, and you're currently a data analyst. Can you share some of the different ways you've had to communicate your work to others and which ones you find yourself doing more? So, as a data analyst, well, as any sort of data professional communicating data is one of those things that you just can't avoid. You have to do it. Otherwise there's no point, if you're not sharing your results, then what are you doing. So, I've had to communicate data, I would say primarily in two different ways, either through visuals or just through an oral explanation. And they each have, you know, their own time and place. Because every data story has different presentation needs. It's never a one size fits all. And more often than not, I choose to present my data through visuals, you know, like a dashboard or a full on presentation, either in my schoolwork or in my work work. And I would say that again, more often than not, I try to combine visuals and an oral presentation, right. Because you also don't want to overwhelm your audience with everything in front of them. So doing different visualizations, pie charts only when necessary, and combining that with explanations. In a way to provide the audience with different methods of interpreting the story that I'm trying to tell, right? Because not everybody has the same learning style. So like for me, auditory processing is very difficult. So I try to incorporate different presentations, different tools for the audience to be able to interpret the story that I'm trying to tell. Times where I try to use only oral is if it's a very casual or very simple conversation, just maybe even like a very quick ad hoc requests. Like, Hey, could you explain why very quickly I'll use just like an oral presentation, but for the most part, I try to use oral and visuals in my presentations to communicate data. I really appreciate how you mentioned that people have different learning styles, because I think. This a lot from folks. And I think in the data field, we end up obsessing over only the visualizing part, like data visualization as being our go-to. And yeah, it's many people are visual people. I'm a visual person as well. And I have a harder time like you with processing things through audio. I prefer visuals, but not everyone is like that. So I really appreciate you reminding us. People learn differently and to use a variety of different ways to communicate to people based on who you are speaking to. So thank you for calling that out because I think that's something for us to keep in mind. Have you found yourself that you've had to communicate with written work more like with reports or emails a lot, or is it usually through presentation? Usually I will send an email summary after our presentation. I try not to communicate data in the email or the I am sort of way, because I feel like there could be lots of context or tone but it leaves it too. Open-ended on their end where they could come to a conclusion that may not be what I'm trying to say. And so I will always try to opt for communicating that orally or again visually and then providing the text later as a supplement, rather than sending only text. Cause I don't want anything to be missed out. That totally makes sense. I know this is kind of. Very rare occurrence for data professionals to communicate a whole report or something through IM but have there been instances where you've had maybe like a manager or someone else like pings you, Hey, can you quickly like, remind me what you found with this analysis? What have you done in those situations? So I very frequently, I will get an, IM, I guess people feel like that's a quicker way to communicate and I understand, I'll get an eye. That's like, Hey, why did you say this? Or, Hey, why this and that? And, you know, if it's, if it's a very brief, and it's a very easy explanation, like I could just say, oh, well the doctors were out that day. That's why the volume is so low that I, I don't mind maybe messaging in an, on. If something is a little bit more complex or there's a little bit more context behind it, I will try to request a meeting or I'll, you know, give a subtle hint, like, oh my calendar's up to date. So if you'd like to schedule some time to discuss it, I would be more than happy to just because I'm very afraid of like someone misunderstanding what I'm trying to say. And I definitely want to make sure that they're getting the full picture. I know a lot of people want quick responses. They want like the high level one liner summary, and sometimes the is not so simple. And I think that's something that's a balance we need to do. Right. As a data professional is like being able to communicate. Accurately and effectively, but also in an efficient manner. So yeah, it's a balancing act. So I appreciate you mentioning this tip because I know there's many of us or those who are listening, who are looking into getting into the world of data, that things are not going to be. Very smooth sailing in terms of communication. It's not going to be one presentation and everyone gets it right away and there's no more follow-ups Oh, never. get a route. There's going to be these kinds of other ways of communicating. You'll have to also master and you won't be good at it at the beginning necessarily, but you get better at it with experience. I think. Yeah, it's also very dependent on the audience. Especially if you have like a new team member onboarding, then you have to completely learn how they prefer to communicate and how they interpret things. And so it's always a learning curve, but it definitely becomes easier. The more you do it. Yeah, definitely. And as a data professional, what pain points have you noticed other data analysts experience when they're first starting off in their job? And what advice would you give them. I would definitely say one of the pain points that I noticed for people as they begin their data career of lot is that they are hesitant to come to a conclusion. And that drives me crazy cause I'm like, that's what we're here to do. We're here to come to a conclusion. So what I see all the time is that people will make all the graphs, so write beautiful code. They'll have everything set up and it's amazing. But when it comes down to interpreting their results, they freeze up and I find that it's more than likely due to people, not feeling confident or empowered to make those decisions. Especially as a woman, sometimes I feel. I'm not empowered. Like who am I to decide that? Right? Like I'm just an analyst. But that's literally what we do. That's the whole point of doing the whole analysis. Eventually you have to tell them, you know, what comes next. That's always going to be the question, what do we do now? And so my advice to them would be to relax and to believe in yourself, being confident in yourself can take you from. Zero to a hundred, like it really sets the bar for you to be able to do the things that you're trying to do. And one of the things that I hope people are trying to do is to be, you know, the best version of themselves. And so, you know, it's, you're, if you're looking at the graph, if you've already done the graph, if you've already done the full analysis, the results are right in front of you. If the Northwest region is showing that they have the highest sales say that if the best month for sales was October you're. Say that if the data isn't conclusive and I feel like a lot of people are afraid of the data is inconclusive to say anything. Like they want to say something. But saying that it's inconclusive is something, no data is still data. So, definitely be confident in yourself. You were hired for a reason, you know, do what you're there to do, make a conclusion. And if somebody doesn't agree with you, then you know, that's another part of the process. But. That would be my advice. That is really great advice because I was that person when I was starting out, I had a hard time giving recommendations from my own work, from my analysis. And I remember my boss being frustrated is like, well, what's next? Like what you were saying? You know, what's next? W what, what should we do? And. I was not confident at all in my ability to be able to give recommendations. So I really liked this advice. And I think it's true. We have a little bit of imposter syndrome and it could be, you know, because we're a woman, but I am also from a non-traditional background. So I had major imposter syndrome when I was starting out. It took me a while to get comfortable with giving recommendations and actually confidently presenting conclusions and insights from my data. If there's anyone listening here, who's also experiencing the same thing. Just try to believe in yourself, have confidence in yourself and know that, you know, you're, you're in this place for a reason. People thought you were capable in doing your job. So, and at the end of the day, what people want from you, they do want you to tell them what to do next. And the other advice that you gave about inconclusive research. I've been in that position a few times too, and I know it's not the best or the sexiest story to tell people is like, well, their analysis was inconclusive or the data is useless. You know, that's not what anyone wants to hear, but sometimes that is a story you need to tell. And that is a story still. Right. You have to be artists that if it's inconclusive or if the data just was not great, there is still an action point from there. You need to, you know, maybe build a better infrastructure for collecting the data so that you can go back or maybe, you know, a year from now, you want to revisit and you'll have better data and you'll be able to come to a conclusion. It's better to be honest and to come up with a conclusion that doesn't match the analysis that you made. Yeah, exactly. Now for this next segment, I want us to pretend like you are presenting to a non-technical manager about a recent data project you completed and you're sharing your findings with them. So at this point you're not stuck or anything, you're not asking them for help or for any input on your data work, you are ready to present your findings with a confidence that we just talked about. Right? So I'll say a data term and you'll have three options to choose. Option one. Should you include this data term in your presentation as is without defining it? So just say the data term, just slip it in there. Like it's natural and they know what you're saying. Option two is to skip, including the data term altogether. Maybe not even. And the last option is to try to translate or explain the term in a way that a non-technical person would be able to understand it or say it in a way that's relevant for them. I am curious to hear what Katie thinks and also what you listeners think too, because you know, there's no right or wrong answer, especially since I've given such vague directions about it's a non-technical manager, but you know, or non-technical person, but this person could actually, when you're actually presenting to them, they could actually be educated in these terms. So we'll get started with the first term P value. Okay. So for the P value, I would choose to translate it. And this might be controversial, just my opinion but essentially the P value determines if the metrics impact was either not random or possibly random. We never accept the null hypothesis, we always either fail to reject or reject. So either it was not random or it was possibly random. And the reason why I would translate this term is because the. Other version of the P value, like the other, I guess, word would be statistically significant. And I avoid using that term and we'll go over why later, but short answer, I would translate it. And then I would define it as whether or not the metric was impactful, randomly or not random. You mentioned statistically significant or statistical significance, and that often does come up in conversation and it is something that has a different meaning in the non data or non statistical world. So I'm curious to hear what you would say for that. So for statistical significance, I would skip it. And it's for the exact reason that you mentioned, I find that statistical significance means something a little bit more like seriously contextually. And the data world or in the statistics world, then the word significance does and the common language and a non-technical person might hear statistical significance and zone in on significant and not really understand the full weight of the word due to the fact that we use significant so casually. And I'm so guilty. I'll always be like, yeah, Cigna. It was significant. And I don't actually mean statistically significant. I didn't do the math. So that's the reason why I try to avoid it. That's a really good point. And I have actually come across people who define significance differently because they're not thinking in terms of this to statistical significance and that does get lost in translation. And that can actually impact the, how your message is interpreted. So I would agree and be cautious about using that. The next term correlations. So I always leave correlation as is a lot of people know what correlation is outside, outside of the data world, because it is also used colloquially, if you will. I don't really think that there's any like noticeable difference in the nuance between the way that it's used casually and the way that it's used in data. And so I think that it's fine to leave it as this. And the next term, how about outlier? What would you do with that? So I would leave it as is for the same reason as I would leave correlation as is, I feel like colloquially and within statistics, it means kind of the same thing. We use outliers to like define ourselves as members of our family, like the black sheep of the family. So I would say that a non-technical person, if I use the word outlet, they would understand what I meant without me having to. Yeah, that makes sense. Would you want to. explain to people how you, I know how people identify outliers can vary, would you want to go over that with your non-technical audience members? Like how you came about identifying these out. If I felt that it was relevant, I might I'm not a fan of removing outliers unless it was. Unless you were, it was correct to do so. So if the outliers are there, I might go into how I found them. It also depends on how long you have for our presentation. Right? If you have 15 minutes, I might not spend so long talking about my process as much as I would. My results. Yeah. Makes sense. All right. So the final term is residual Yes. So I would translate this term because in my opinion, it's too important in the understanding of the results that it can't be skipped. We have to talk about the error. And it is very easy to explain, to put in like the simplest terms. But I also wouldn't leave it as is because if you see residual error, like, I don't know what residual means off the top of my head. So. Or error. That sounds scary. Right? So somebody might see that and start to get nervous. So I would translate it. And I basically, I would tell them it's the measure of the accuracy because ultimately that's what it is. It just tells us how accurate the model was. So yeah, I would translate it. I would include it. It's important. And I don't want people to be scared. And sometimes residual errors can actually help you improve your model. And so maybe when you're presenting to your audience members, you're actually telling them a story about how you need to do another iteration or how you're talking about a previous iteration of your model and why you actually had to reject it and actually work on improving it. So it could be part of the data story too. But I agree about not saying it as is because I think the word error has a negative connotation and a scary connotation for a lot of people. So. Right. Absolutely. Well, thank you so much for playing this game. I really think that you captured all of the really great statistical terms and are they really relevant ones? And I really appreciate that they were all statistics. I was like, wow. She really gets me. Yeah, I I'm having a math person on here. I'm going to bring it all these terms. So the final question we have for you, Katie, before you leave this show is I heard you started a new website. And can you tell the listeners a little bit more about this website and what viewers can expect to find it? Yeah. So I actually started a website. I was inspired by Hana because their website is beautiful. My website is, uh, data. So you uhhdata.com. And there you'll find. A couple of pages. So you'll find a little bit more about me, my journey as a data analyst a link to all my socials. So you could follow me on Instagram, on TikTok Twitch, sometimes I stream. And then I have a page full of all sorts of different resources for all the different tools that a data analyst might use, or that I have used in my journey. And still to this day use. Including links to some of my favorite social media accounts for daily inspo, like Hana and some of my other favorite social media posters. And then in the future, hopefully some cool data related apparel. That's great. I'm so happy that you're doing this and thank you so much for providing all these resources for the data community on your website. So I will link the website in my show notes. For those listening, you can find the website linked in the show notes for this episode and. Your social media handles that you used to be a data. So is this, are you going to be going back to that or what are you going to keep your new social media handle? So actually not to sound like too much of a fan because I really am a number one HANA Khan fan. I, when I noticed that you were doing trending analytics for like your brand and then like how hanalytx was like a sub-part of your brand. I was like, that's so smart because I eventually would like to branch out. I would eventually maybe like to host. Other curators, other content creators under my brand and, and they would go under their own content creation name social media name. So I wanted to have something that was sort of an umbrella for the brand and then leave me room to grow into what this will become as I go. That's awesome. I'm really excited for you. I'm really excited about this website and the things that are coming up with this website. So people definitely make sure you're keeping an eye on the website book market, and then also follow her on social media. They want to share your social media handles here on the. Yes. So I am Katie dot DBS, the letter K T dot DBS on Instagram and TechTalk. And then on Twitch, I can't have the dots. So I'm Katie DBS. Well, thank you so much, Katie, for coming on here today and for educating us, you are definitely welcome back any time too. I mean, I would love for, to have you again here and we can talk more about math. We can talk more about data I know there's going to be some stuff you're launching soon. So I don't know if I should, if I'm allowed to say it. Well, I don't want to put the hopes out, but I do. I know what you're referring to and it will be out hopefully very soon. I just want to do a really good job on it. I know you will. Thank you so much, again, I hope you enjoy this interview with Katie. I particularly enjoyed the segment where we played the game with the data terms. I recognize that your audience will be different than the hypothetical one I presented to Katie. So you don't have to exactly follow what we suggested here, but I hope it gives you something to consider about each of these terms the next time you think you may have to present one or more of them to your non-technical or non data on members? I would love to hear thoughts. And if there are other data terms, you have questions about, you can email me and I can include them in a future podcast.